It’s a small world, after all
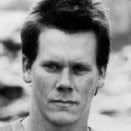
Many of you have probably heard of the “Six degrees of Kevin Bacon”game. This is based on the work of Stanley Milgram beginning in the 1960s, and brought up again more recently in a 1998 Nature paper, "Collective Dynamics of ‘Small-World’ Networks,” by mathematicians Watts and Strogatz. Milgram conducted a number of studies using his “lost letter technique,” in which letters were sent out and then needed to be forwarded onto reach their destination. In one instance, Milgram sent out 160 letters to individuals in the midwest, with instructions to pass them along to acquaintances who would be most likely to reach his stockbroker friend back east. Almost all of the letters that reached the stockbroker did so via one of 3 friends, and most did it within 6 steps--hence the “six degrees of separation” idea.

Another “small world” phenomenon is disease transmission. Social architecture affects, and explains, factors that impact the spread of disease—the people we are in contact with, and in the cases of sexually transmitted diseases, the people we sleep with. At the base of this phenomenon in infectious diseases is an idea called the "tipping point." This is something epidemiologists have discussed much in recent months regarding the potential of an avian influenza pandemic—tiny influences can have startling effects. A simple mutation may make a virus more easily transmitted between humans. Given the right environment, this may allow the virus to sweep through a population, causing a world-wide outbreak. Under different conditions, it may cause a few infections and fade away; a few factors may make all the difference. Mathematical modelers can examine these factors in computer simulations in order to determine if a disease will burn out, remain endemic in a population, or become an epidemic. A critical determining factor affecting these outcomes is the basic reproductive rate of a disease (R
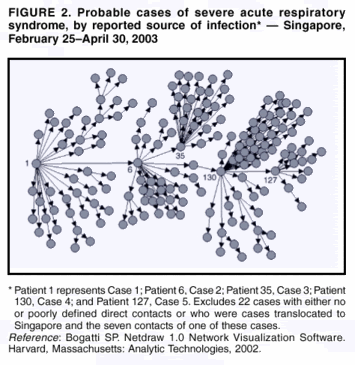
It takes careful epidemiological analysis to identify these superspreaders, however. One limitation of epidemiological analysis is that populations are generally divided into homogeneous subgroups--people of the same age, or race, or occupation, etc. This makes it possible to carry out studies comparing groups of individuals, but at the same time, it creates false divisions; life is always messier. A new paper published in Nature seeks to investigate some of this "mess," addressing the heterogeneity of transmission number among individuals and its effect on spread of disease. This new study by Lloyd-Smith et al., instead of using R
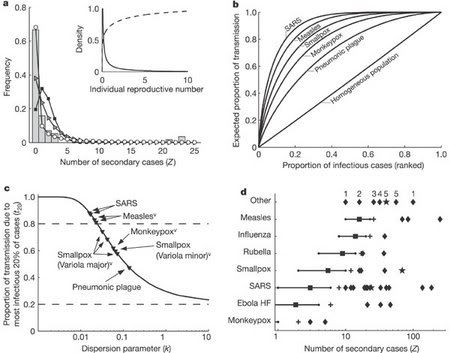
To sum up their findings, they suggest that disease with a high variation in ν show infrequent, but explosive, epidemics after introduction of a single case, a statement again borne out by an examination of the epidemiology of SARS, a disease which showed a high variation in ν. Indeed, many cities were exposed to SARS, but very few had explosive outbreaks. Is this universal? It will take more careful studies to determine that, but it suggests a new avenue of investigation.
Indeed, knowledge of the architecture of these outbreaks has immediate real-world applications. If the superspreaders could be identified early in an outbreak, interventions (vaccination, isolation/quarantine, treatment) could be targeted to this group. For example, another recent report highlights this same conclusion for malaria: identifying and targeting the superspreaders could reduce the disease burden enormously. Additionally, this work suggests, again, that we need better surveillance in order to detect these events while they are in progress. We can’t control superspreaders that we aren’t aware of, or only identify retrospectively, weeks, months, or years down the line.
Finally, this work—and indeed, much of the work done in the field of network theory--also points out, again, how things that appear to be “designed” can be the product of chance and circumstance. Each of these “small worlds” reveals a very similar architectural design. Each is dominated by hubs—a few extremely well-connected individuals, or web sites, etc. Additionally, this is not merely a qualitative difference: instead, they have a specific mathematical signature, which is nearly identical from one network to the next. I won’t go into the details, but Mark Buchanan has written a very layman-friendly book on the subject. (To be complete, Watts and Strogatz have also written books on the subject, but I've not read them yet). In Nexus, Buchanan notes:
What we see then is a kind of natural order that for mysterious reasons seems to well up in networks of all kinds and that does so despite the complexities of their individual histories….In biology, Charles Darwin’s idea of evolution by natural selection offers an extremely powerful organizing framework within which historical accidents take place. In the context of networks, there must also be some deeper principle at work.Along a similar line of thought, Nobelist Herbert Simon once said, “The purpose of science is to find meaningful simplicity in the midst of disorderly complexity.” Network theory has shown how this can be done, and unlike “intelligent design,” has immediate practical applications in a vast range of fields.